2.1 Defining and categorising research data
Have you ever paused and reflected on the term "data" and what it actually means? If confronted with the question, many of us will probably list concrete examples of data types, for instance interviews and different types of measurements,
or computational models. And this is fine, and is in fact included in the definition of data in many official documents, such as institutional data policies. But what actually makes an interview an example of “data” and not just a conversation
between a person asking questions and another person answering these questions? What makes the temperature outside, at a given moment in January, a “measurement”, and not simply a sensation that makes water and noses freeze?
Christine L.
Borgman, Distinguished Research Professor in Information Studies at the University of California, LA, has written an excellent book entitled
Big Data, Little Data, No Data: Scholarship in the Networked World, published in 2015, where she addresses
the question of how to define data.
In her view, data can be seen as “representations of observations, objects, or other entities used as evidence of phenomena for the purposes of research or scholarship” (p. 28), and these entities “become data only when someone uses them
as evidence of a phenomenon” (p. 28). One example she uses to illustrate this is the old family photo album that becomes data for a researcher who studies clothing styles from a certain period in time.
According to Borgman, it may nevertheless
be constructive to discuss the concept of data along tangible dimensions.
- Operational definition: Data is a concept without strict boundaries, and as society and science evolve, new types of data emerge in the data landscape. Nevertheless, to handle data collections it is necessary to be aware of which type
of data we are working with, as the different types may require specific management procedures. Organisations that work on archiving systems and standards for data description do not necessarily provide exhaustive lists of what data may or
may not be. Rather, they provide the metadata standards necessary to identify and describe types and sources of data in a sustainable manner.
- Categorical definition I: Data can be categorised according to their level of processing. One very illustrative example comes from NASA's Earth Observing System Data and Information System (EOSDIS), where data processing ranges
from
Level 0 to Level 4. On Level 0, we find raw data with full instrument resolution, while on Level 4, we
find models or results from analyses of data on lower levels. Information about level of processing is necessary for operators of data collections, as it may influence how data are curated and adapted for reuse.
- Categorical definition II: Data can be categorised according to their origin, whether they come from observation, computation or experiments (see details below). Understanding the origin of data is important for decisions regarding future curation and preservation. Studies based on observational data may be hard to replicate because they are associated with specific times or places. Studies based on experiments or computations are more easy to test through replication studies, if they carry good documentation to enable the studies to be repeated. To complete the overview, Borgman mentions that data also may originate from other records, such as archival material or governmental documents.
Replicable and reproducible studies
The findings in research studies can be tested by replicating a study. This means to do a new study with new data, testing the same hypothesis, to see whether one reaches the same conclusions as in the original study. This is an important part of scientific progress. It is vital to test if previous conclusions are to be considered valid or if they are weakened and in need of more research.
A study can also be tested to see if it is reproducible or not. This is done by accessing the data and a detailed description of the method of the study, enabling a validation of the analysis and interpretation of the data. And then comparing to see if the conclusions of the original study are sustained.
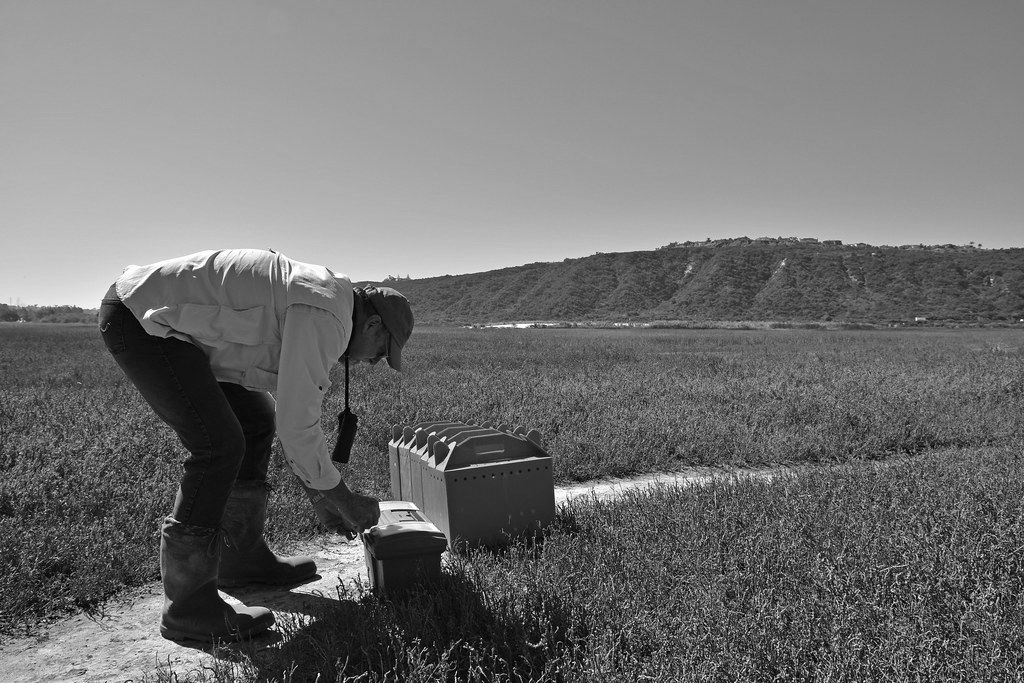
Fieldwork
Photo:
"The birds were transported from Living Coast Discovery Center to Carlsbad in these blue boxes"
by
USFWS Pacific Southwest Region is licensed under
CC PDM 1.0
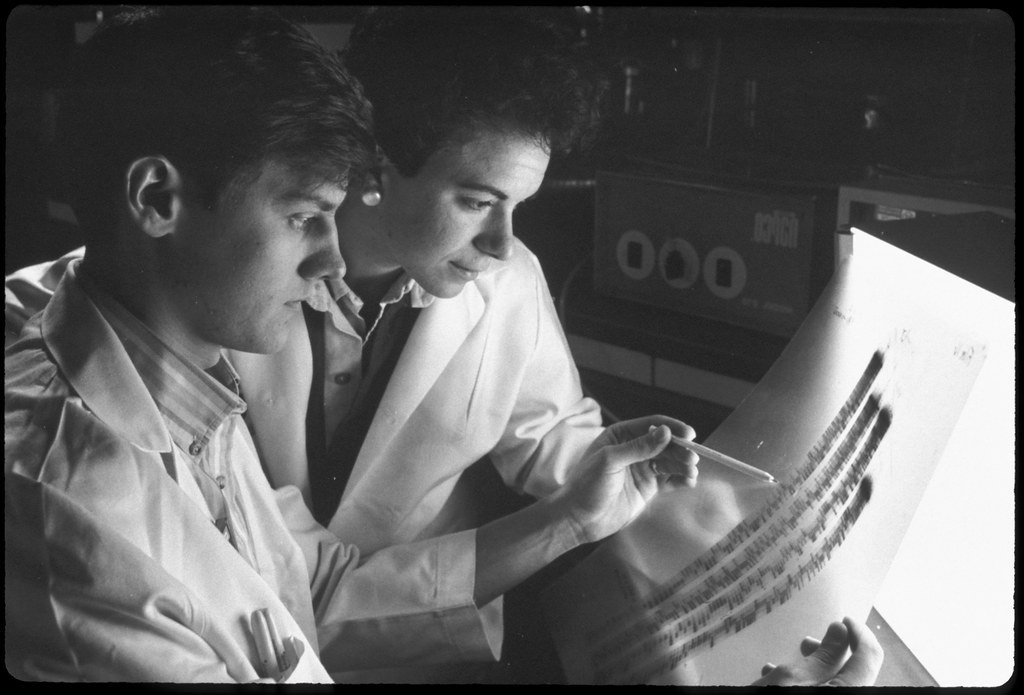
Lab work
Photo:
"Graduate Students--Research Data"
by
Uniformed Services University is licensed under
CC BY-NC-ND 2.0
Lessons learned
- Observational data result from recognising, noting, or recording facts or occurrences of phenomena, usually with instruments.
- Computational data result from executing computer models, simulations, or workflows.
- Experimental data result from procedures in controlled conditions, to test or establish hypotheses or to discover or test new laws.
References
Borgman, C. (2015). Big Data, Little Data, No Data, Scholarshop in the networked world . MIT Press.